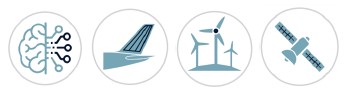
Manufacturing in the EU is crucial, given that it is one of the main drivers in innovation, job creation and sustainable growth. It involves almost 2,000,000 companies that provide approximately 28.5 million jobs. Therefore, its impact is significant in terms of economic share, with it being ~18% of the EU-27 gross domestic product. However, at present the dependence of processing on external factors or disturbances is still important. With that in mind, the cumulative and recurrent costs for the non-implementation of the appropriate correcting on-the-fly manufacturing policies that could allow recovery from failure will produce, without any doubt, negative impacts on the competitiveness of the EU industrial sector in the future.
IMDEA Materials has developed efficient Artificial Intelligence (AI) methods to automatically detect defects produced during manufacturing of structural composites by liquid moulding by using Virtual Processing (VP) techniques which include [1]:
• Creation of synthetic databases obtained by means of computational mechanics (fluid and solid) for typical manufacturing processes. This includes the use of a comprehensive multiscale and multiphysics VP simulation environment.
• The data generated is used to deliver regression-classification tools for the automated detection of processing disturbances from processing sensor signals.
• Adequate quantification of uncertainty of processing model parameters including model inadequacies.
• A laboratory-scale demonstrator (Fig. 1) where the AI tools for automated detection of defects is being deployed. A network of pressure and temperature sensors are distributed on the demonstrator and their corresponding signals used to detect and quantify the presence of a manufacturing disturbance that may produce a defect.
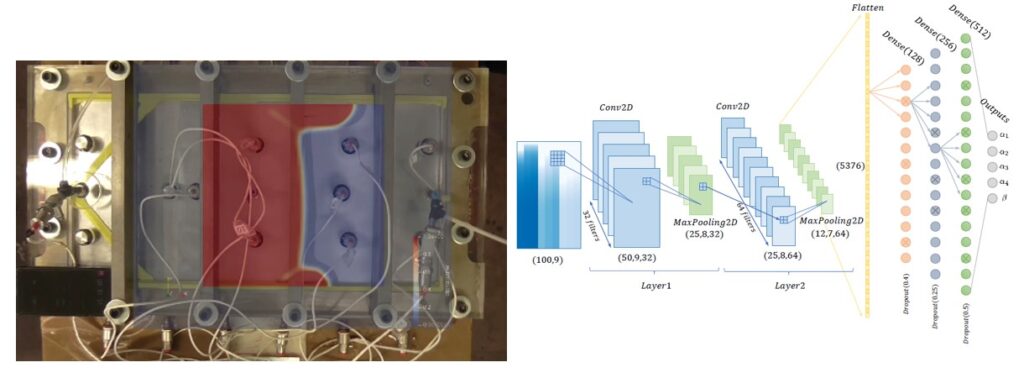
IMDEA Materials expertise includes the capabilities produce advanced VP modelling of manufacturing process, lab-scale experimental set-up including sensors and actuators, and expertise to develop deep-learning methods.
For more information about this technology, reach us at proyectos.materiales@imdea.org.
[1] C. González, J. Fernández-León, A Machine Learning Model to Detect Flow Disturbances during Manufacturing of Composites by Liquid Moulding, Journal of Composite Science, 4, 71 (2020)